Last Updated on February 27, 2025 by Editor
AI has changed drastically, evolving from an idea to an essential tool today. This article explores how a 50-year journey has shaped business, changed views on man-machine relationships, and created both opportunities and challenges. This article will be helpful to you if you want to learn more about AI, learn the key steps in this technology’s development, and find out about current and potential uses for AI.
The Early Beginnings of AI
The concept of making machines that can reason and learn like human beings cannot be considered entirely contemporary. However, the origin of AI can be traced further back in history than that.
The Conceptual Foundations in Antiquity
As early as prehistoric times when people did not even invent the terms “robot” or “artificial intelligence,” ancient man dreamt of producing intelligent beings. Myths and legends concern personification as well, as the Greek myth of Pygmalion or the mechanical servant of wind, Talos.
The Birth of Modern AI in the 20th Century
Actually, the scientific recognition of AI started in the early part of the twentieth century, although it was significantly influenced by developments in mathematics, symbolic logic, and computing.
- Alan Turing: Around 1950, the British mathematician and logician Alan Turing devised a test, now named after him the Turing Test, to measure whether a machine with intelligence to a human level was fully possible. Turing was preeminent in several of ways, which is why he is overtly often referred to AI as its progenitor.
- The Dartmouth Conference (1956): Also widely regarded to be the real starting point of AI as a scientific discipline, this conference was initiated by John McCarthy who first used the term AI (Artificial Intelligence). The conference is an indication of the direction of a new investigation into building equipment that has the capability of “thinking.”
Key Milestones in AI Development
Over the years, there have been several achievements that have taken place in the journey to what is known as present-day AI.
The Rise and Fall of Early AI (1950s-1970s)
Very strong early AI research, also referred to as Good Old-Fashioned AI (GOFAI), is symbolic AI and relies upon symbolic representations of domains and logic-based algorithms. This approach had many challenges, however.
- The AI Winter: They were quickly succeeded by periods of disillusion in the 1970s and the 1980s when progress gave way to stagnation. Recent advancements in artificial intelligence were not as fast as expected; funding was significantly reduced; people lost interest in what is now called the AI Winter. This was because initially created AI programs were incapable of dealing with realistic application-based problems and contexts.
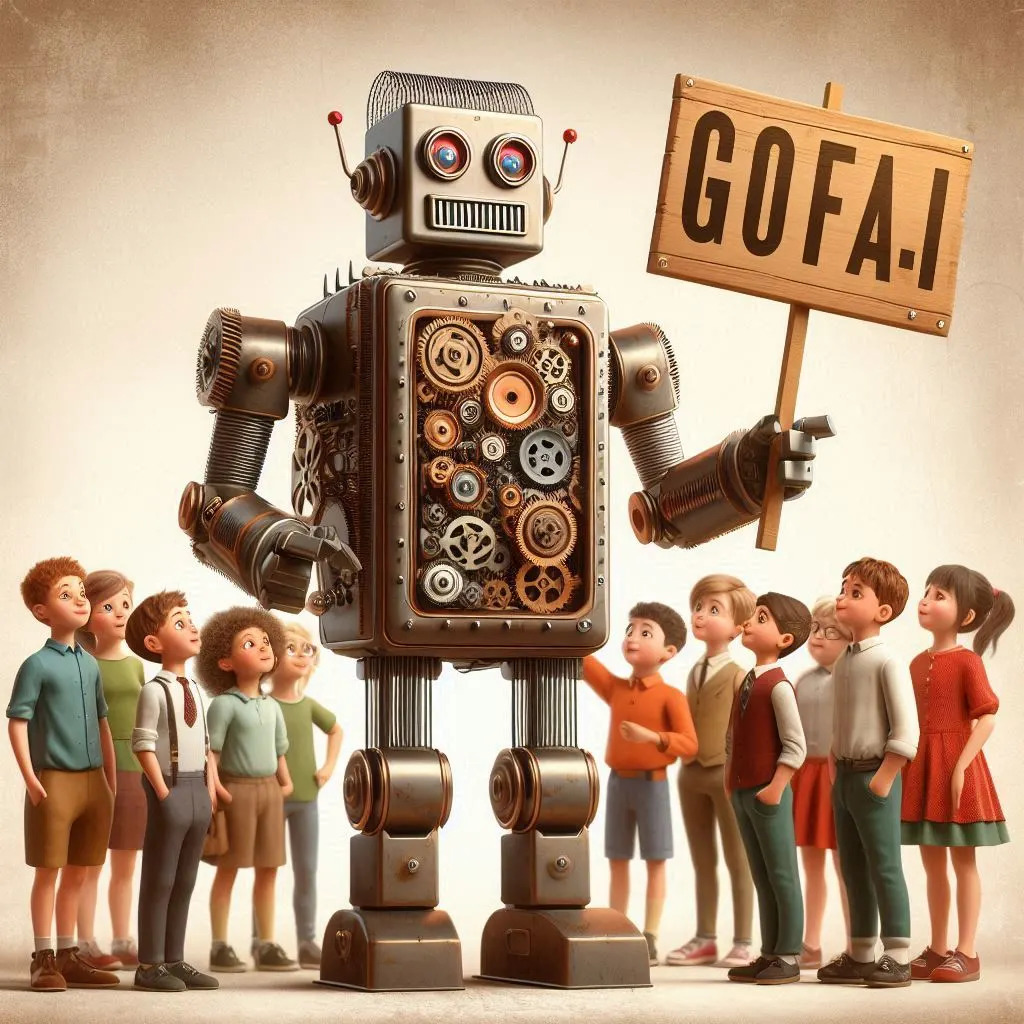
The Emergence of Machine Learning (1980s-1990s)
Machine learning became a major advance in the field in the early 1980’s and early 1990’s. Learning from data replaced hand coding rules into the machine, where we can get an extra edge in how we learn.
- Neural Networks: Skills such as the human brain were needed to train machines to learn patterns in data. However, the practical use of these calculations and data was limited because of the weak calculations and insufficient data.
- Deep Blue vs. Garry Kasparov (1997): In so many ways, IBM’s Deep Blue defeating world chess champion Garry Kasparov was one of the first major public victories for AI. This was a big one in terms of the stuff that Ai is able to work on that is complex, that’s strategic.
AI’s Breakthroughs in the 21st Century
At the dawn of the 21st century, big compute, big data, and better algorithms took AI to a golden age.
- Big Data Revolution: The digital era brought with it the massive generation of data that AI systems needed to train on. When huge data were processed and learned via machine learning models, particularly deep learning, they thrived.
- Speech and Image Recognition: Speech recognition (e.g., Apple’s Siri, Google Assistant), and image recognition (e.g. facial recognition systems), became a reality with technologies. However, the realization is that AI has the potential to see and interpret the world we are in.
- Google’s AlphaGo (2016): When Google’s AlphaGo beat the world Go champion, Lee Sedol, this was a major leap. Go, unlike chess, requires wide sweeping names of moves, and has always been deemed one of the toughest things for AI to accomplish: Defeating a human expert. To capture such complexity, AI systems that could take on difficult, abstract problems had arrived in the form of AlphaGo’s success.
AI Today: From Narrow AI to General AI
AI is projected to contribute $15.7 trillion to the global economy by 2030.
Approximately 35% of businesses have adopted AI technologies, and 77% of devices currently in use feature some form of AI.
Today, AI is almost in every corner, it is used in healthcare, financial systems, education systems, and entertainment. It exists in two primary forms: Narrow AI and General AI.
Narrow AI: The Current State
Almost all of the AI we are currently surrounded with could be classified as Narrow AI or “Weak AI”. Such systems are optimized for some narrow tasks to be accomplished at a greatly high rate, but they are not encompassed by a full-scope intelligence to solve as many tasks in various fields.
- Autonomous Vehicles: Autonomous vehicles, such as the ones produced by Tesla and Waymo, operate with the help of Narrow AI in order to capture their environment and make decisions for the car on the fly.
- Natural Language Processing (NLP): Current fancies such as OpenAI’s GPT models or Google’s BERT have taken the knowledge of NLP to the next level where machines can understand, generate, and reply to human language correctly. By this NLP is evident in the use of chatbots, voice assistants, or even the use of AI-generated writers.
General AI: The Elusive Goal
About 85.1% of AI users utilize the technology for article writing and content creation, indicating a significant shift in the content production landscape.
General AI is also referred to as “Strong AI” and applies intelligence to any task, as a human being, can. However, as it is one of the themes of science fiction, we are not yet close to creating General AI.
- Challenges: The principal problem in attaining General AI is to provide machines with another form of reasoning, flexibility, and sense of context like human intelligence.
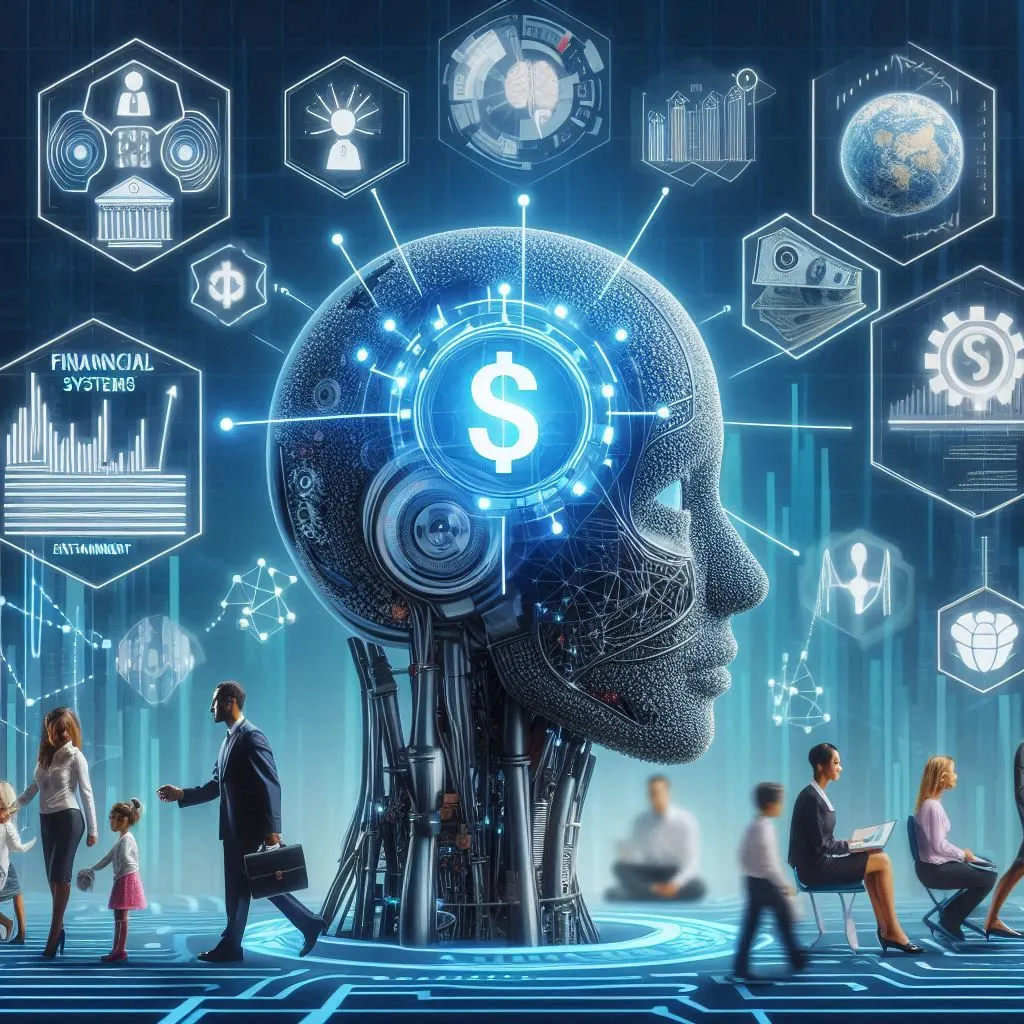
The Future of AI: Ethical Considerations and Opportunities
As AI develops, there are so many unexplored opportunities and at the same time, so much work to be done around ethics and societal challenges.
Ethical and Societal Impacts
50% of consumers express optimism about AI, while 33% believe they are using AI platforms, although actual usage is reported at 77%.
AI is revolutionizing industries and can be used as a tool to enhance the well-being of citizens in nations across the world; however, it has created an ethical dilemma.
- Bias in AI: The findings also suggest that AI systems including deep learning models, may replicate biases inherent in the data on which they are trained. When left uncontrolled these prejudices may manifest themselves in prejudiced decisions in issues involving employment, policing, and financing.
- Job Displacement: Due to AI, automation may take up millions of occupations especially those dealing with routine tasks. This has raised questions on the future of work, the future of jobs, and the future of workers needing to be reskilled.
By 2025, AI is expected to eliminate 85 million jobs, but create 97 million new ones, resulting in a net gain of 12 million jobs. A survey indicated that 32.9% of businesses have replaced some human tasks with AI solutions, with content writing being particularly affected.
Opportunities and Benefits
The opportunities provided by AI are vast, particularly in fields like healthcare, where AI-driven diagnostics and treatment planning can save lives.
- AI in Healthcare: Machine learning models are being created in healthcare to diagnose radiology images, estimate patients’ prognoses, and even in drug development to help make healthcare smarter and more precise.
By 2030, it is estimated that intelligent robots may automate up to 10% of nursing tasks.
- Climate Change: AI is useful in fighting climate change through efficient use of energy, prediction of the changes, and even change of agricultural practices.
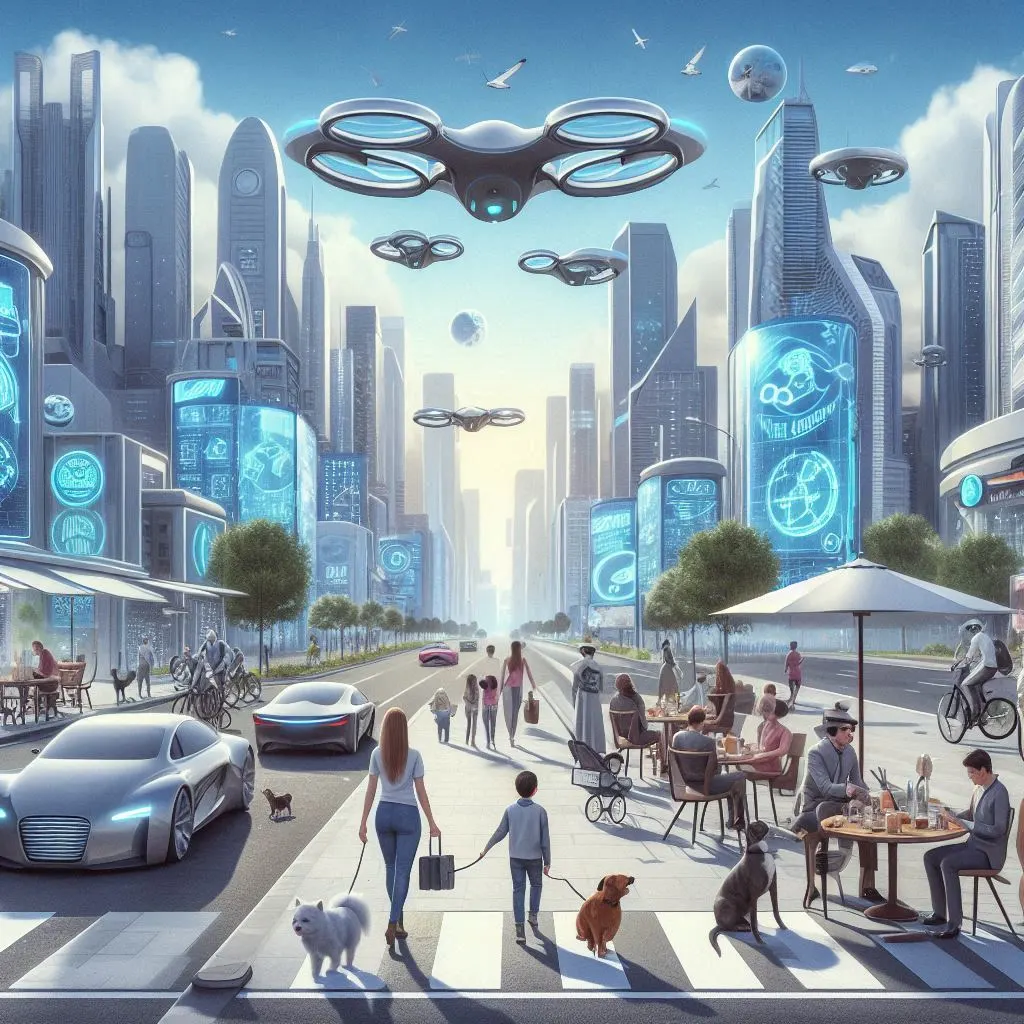
Conclusion
AI can be best explained as a narrative of grand aspiration, failure, and success. Starting from its birth as a thought experiment to its current position as a tool for redefining entire industries, AI remains at the forefront of what can be done by machines. AI brings the promise of virtually any future we may desire, though the promise should come with the caution of understanding the correspondingly inexhaustible supply of ethical and social ramifications. The future development of AI will rely not only on the future invention of new inventive technologies but in the way that these technologies will be implemented.
Sources and Further Reading: AI Trends, Tools, and Insights:
By 2030, AI will contribute $15 trillion to the global economy
22 Top AI Statistics And Trends In 2024
The state of AI in early 2024: Gen AI adoption spikes and starts to generate value
16 Helpful Use Cases of AI in Content Creation
25 stats about AI in customer experience that show how consumers really feel